Some Of Problems and Solutions - SDSU Extension

AND Solution
Building Technologies & Solutions - Suppliers - Johnson Things To Know Before You Get This
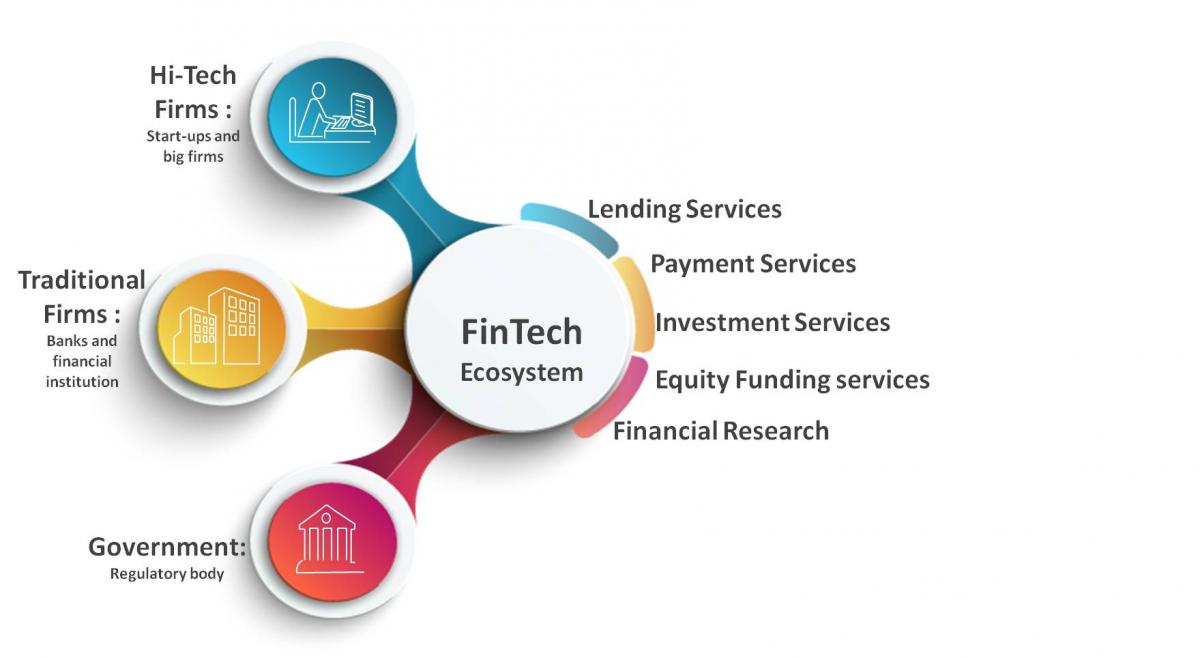
In a lot of cases, bandits are even the ideal techniques. Outlaws need less information to figure out which model is the finest, and at the exact same time, minimize opportunity expense as they path traffic to the much better model faster. In this experiment by Google's Greg Rafferty, See conversations on outlaws at Linked, In, Netflix, Facebook, Dropbox, and Stitch Repair.
Requirements To do bandits for model assessment, your system needs the following 3 requirements.: you need to get feedback on whether a forecast made by a design is excellent or not to compute the designs' existing efficiency. The feedback is utilized to extracted labels for forecasts. Examples of tasks with brief feedback loops tasks where labels can be identified from users' feedback like in recommendations if users click a suggestion, the recommendation is presumed to be excellent.
10 Common Naptime Problems and Solutions - Parents
If the loops are long, it's still possible to do bandits, but it'll take longer to upgrade a design's efficiency after it's made a suggestion. Due to the fact that of these requirements, outlaws are a lot harder to implement than A/B testing. Therefore, not widely utilized in the industry other than at a couple of big tech business.
g. prediction precision) of each design, contextual outlaws are to identify the payment of each action. When it comes to suggestions, an action is an item to show to users, and the payout is how most likely a user will click it.: some individuals also call outlaws for model examination "contextual bandits".
The Ultimate Guide To Connected Services and Solutions, for tires and beyond - The
To illustrate this, consider a recommendation system for 10,000 products. Each time, you can recommend 10 products to users. The 10 revealed products get users' feedback on them (click or not click). However online shopping solutions ltd won't get feedback on the other 9,990 products. If you keep showing users just the items they most likely click on, you'll get stuck in a feedback loop, showing just popular products and will never get feedback on less popular products.
Contextual bandits are well-researched and have actually been shown to improve designs' efficiency significantly (see reports by Twitter, Google). Nevertheless, contextual bandits are even more difficult to carry out than design outlaws, considering that the exploration strategy depends on the ML model's architecture (e. g. whether it's a choice tree or a neural network), which makes it less generalizable across usage cases.